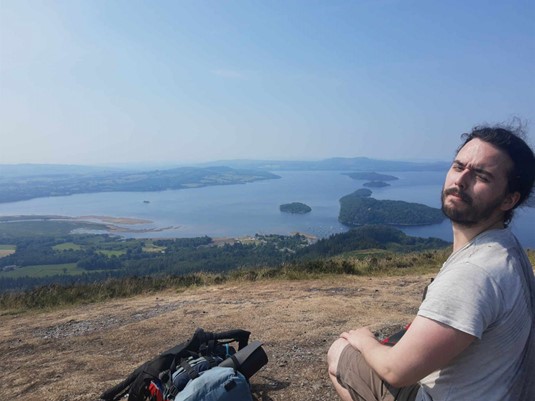
Academic Year | 2023-2024 |
r01mt23@abdn.ac.uk | |
Institution | University of Aberdeen |
Biography
School: School of Biological Sciences
Project: Harnessing the power of AI for biodiversity forecasting
Supervisors: Roslyn Henry, Georgios Leontidis, Justin Travis & David Burslem
Undergraduate Education: BSc Zoology, University of Aberdeen; BSc Computer Science, Manchester Metropolitan University
Postgraduate Education: N/A
Research: Land use and climate changes, induced by anthropogenic activity, pose major threats to many species and are significantly responsible for the extinction of many others, resulting in particularly devastating impacts on biodiversity. To protect extant species from these threats and to develop effective conservation initiatives, modelling the impact of future environmental changes on species is key, though gaps in our knowledge of species traits (morphology, physiology, etc) act to significantly diminish our capacity to reliably make such predictions for many species. As carrying out empirical studies for each species-trait is infeasible, both from a time perspective and a financial one, alternative methods of predicting likely values of species traits are necessary.
In my project I will be investigating the potential for artificial intelligence (AI) to make such predictions, and I will then utilise these predicted traits in process-based models such as ‘rangeshifter’ to make predictions regarding the impacts of climate and land use change. Due to the requirements of training AI my focal species/group will be limited to those for which a large repository of data is available, such as those present on ‘TRY plant database’ and ‘mammalbase’. My work will primarily be desk based and focussed on investigating different AI architectures and their suitability for predicting species traits, and optimising my model and AI architecture such that the output traits are accurate. If successful, this Ai has the potential to fill major knowledge gaps that have prevent effective, large-scale predictions of how ecosystems are likely to respond to change, and thus allow effective conservation.